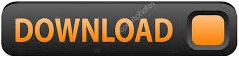

- After effects have particle playground bounce off an object how to#
- After effects have particle playground bounce off an object full#
As such, applications of black-box forward processes often require surrogate gradient estimators such as finite differences or REINFORCE to enable any learning. These simulators are not readily usable for solving inverse problems, due in part to their non-differentiability. This problem has been treated in the vision, graphics, and physics communities, leading to the development of robust forward simulation models and algorithms.
After effects have particle playground bounce off an object full#
Simulation refers to a unified treatment of these two processes.)Įxplicitly modeling the end-to-end dynamics and image formation underlying video observations is challenging, even with access to the full system state. Rendering refers to the interaction of these scene elements - including their material properties - with scene lighting to form image sequences as observed by a virtual camera. ( Dynamics refers to the laws governing the motion and deformation of objects over time. We eliminate the dependence of white box dynamics methods on 3D supervision by coupling explicit (and differentiable) models of scene dynamics with image formation (rendering). Most notably in our context, all of these approaches require precise 3D labels - which are labor-intensive to gather, and infeasible to generate for many systems such as deformable solids or cloth. In contrast, white box methods impose prior knowledge by employing explicit dynamics models, reducing the space of learnable parameters and improving system interpretability. Recently, grey box methods leveraged partial knowledge about the system dynamics to improve performance. These methods require few prior assumptions about the system itself, but lack interpretability due to entangled variational factors or due to the ambiguities in unsupervised learning. Black box methods model the state of a dynamical system (such as the basketball's trajectory in time) as a learned embedding of its states or observations.

The underlying physical attributes of objects and the system dynamics need to be modeled and estimated, all while accounting for the loss of information during 3D to 2D image formation.ĭepending on the assumptions on the scene structre and dynamics, three types of solutions exist: black, grey, or white box.
After effects have particle playground bounce off an object how to#
Imagine watching a short video of a basketball bouncing off the ground and ask: " Can we infer the mass and elasticity of the ball, predict its trajectory, and make informed decisions, e.g., how to pass and shoot?" These seemingly simple questions are extremely challenging to answer even for modern computer vision models. This end-to-end reasoning task requires a fundamental understanding of both the underlying scene dynamics and the imaging process. Moreover, our unified computation graph - spanning from the dynamics and through the rendering process - enables learning in challenging visuomotor control tasks, without relying on state-based (3D) supervision, while obtaining performance competitive to or better than techniques that rely on precise 3D labels.įigure 1: gradSim is a unified differentiable rendering and multiphysics framework that allows solving a range of control and parameter estimation tasks (rigid bodies, deformable solids, and cloth) directly from images/video.Īccurately predicting the dynamics and physical characteristics of objects from image sequences is a long-standing challenge in computer vision. This novel combination enables backpropagation from pixels in a video sequence through to the underlying physical attributes that generated them. We present gradSim, a framework that overcomes the dependence on 3D supervision by leveraging differentiable multiphysics simulation and differentiable rendering to jointly model the evolution of scene dynamics and image formation. Current solutions require precise 3D labels which are labor-intensive to gather, and infeasible to create for many systems such as deformable solids or cloth. Such a system identification problem is fundamentally ill-posed due to the loss of information during image formation. We consider the problem of estimating an object's physical properties such as mass, friction, and elasticity directly from video sequences.
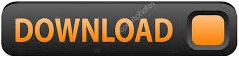